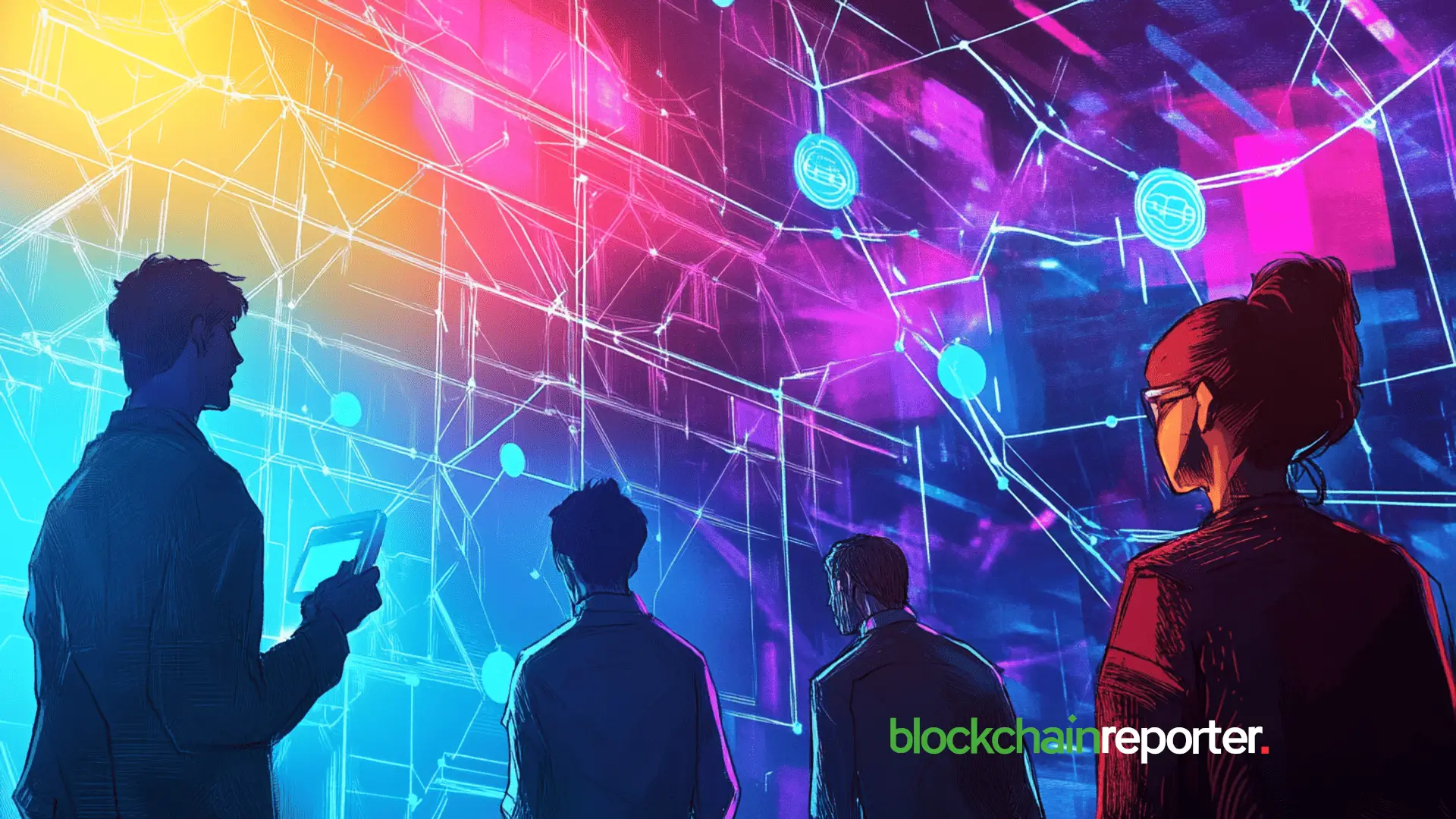
While it’s tempting to think of the AI revolution as being milestone after milestone, a major roadblock continues to hinder further advances: compute availability.
With AI models growing increasingly sophisticated, and demand for GPU resources now outstripping supply, builders face serious difficulties in accessing the computational power they badly need to train and deploy their models. So, what’s a machine learning (ML) engineer to do?
Self-evidently, ignoring the 800lb gorilla isn’t an option: there is no wand that can be waved to eliminate GPU shortages and demand for compute isn’t going anywhere. Fortunately, a solution is starting to gain traction in the world of web3.
The Growing GPU Crisis
To say the global GPU market is experiencing severe supply constraints would be to utter an understatement of epic proportions. Valued at $65 billion in 2024, the market is in the eye of the AI storm as a consequence of GPUs’ role in training deep learning models, making them gold-dust for tech startups, crypto miners, game developers, data scientists, and AI engineers.
Sustained demand for compute power has caused an eye-watering surge in prices, with high-end GPUs like market leader NVIDIA’s A800 commanding prices as high as $36,500. Generally speaking, market dynamics have created a situation where chip costs are running somewhere between 10-15% above the manufacturer’s suggested retail price (MSRP), making AI development increasingly inaccessible for smaller teams and resource-constrained organizations.
High demand and prohibitive costs are just one element of the issue blighting AI development. Centralized cloud providers like AWS, Microsoft Azure, and Google Cloud have also created bottlenecks in compute access, not least because of their struggle to keep pace with escalating demand, leading to extended wait times.
The centralization of compute resources by the likes of Amazon has also caused something of a walled garden to be erected, with access to powerful GPUs often restricted to large, resource-rich enterprises. Of course, centralized cloud providers aren’t the only show in town.
Decentralized Compute On The Rise
In recent years, we have seen the emergence of decentralized GPU compute networks that aggregate underutilized computational resources from diverse sources – and often supply power at a fraction of the cost of AWS and its ilk.
There is much to be said for the decentralized approach, not least the fact that it increases GPU availability and, in the process, promotes energy efficiency. By tapping into previously inaccessible or idle compute power, these blockchain-powered networks broaden access to GPU capacity while driving prices down and creating revenue streams for hardware owners like crypto mining farms and data centers.
io.net has quickly become the most recognizable deployer of this model. A decentralized physical infrastructure network (DePIN) that refers to itself as the ‘Internet of GPUs’, it provides affordable GPU compute by aggregating underused compute from data centers and individuals in 130 countries – a system it says lets it slash costs by up to 90% per teraflop (TFLOP) versus traditional cloud providers.
Built on Solana, a blockchain known for its high throughput (65,000 transactions per second) and robust security, io.net provides access to NVIDIA’s coveted H100 GPUs in under two minutes, enabling AI teams to scale rapidly. Moreover, it supports a wide range of hardware, from 4090s and A100s to CPUs from Apple and AMD, providing enough flexibility to accommodate diverse computational requirements.
Io.net has proven to be a major hit with developers in need of cost-effective compute, particularly owing to its transparency: every job and transaction between supplier and consumer is made visible on-chain. Last year, the company raised $30 million in Series A funding as the value of its native token soared to $1 billion.
The Future of AI Development
By granting developers access to the computational resources they need, distributed networks are carrying the fight to centralized cloud behemoths who have monopolized GPUs for too long. They are also allocating resources more efficiently and cost-effectively, and optimizing energy output into the bargain.
Solving compute bottlenecks is imperative if we wish to unlock the next wave of AI innovation and fix real-world problems. Refreshingly, centralized cloud providers no longer represent the only option for sourcing the GPU power to make that a reality. The question, now, is what happens when all hardware is onboarded to decentralized networks and there is no more idle capacity to tap into.
That’s a question for another day.